Supported by |
|
|
|
Additional cooperation from |
|
|
Seventh International Workshop on Symbolic-Neural Learning (SNL-2023)
June 28-29, 2023
Venue: RIKEN, AIP, Tokyo, Japan
Keynote Talks:
- June 28 (Wednesday), 13:15-14:15
Heng Ji (University of Illinois Urbana-Champaign)
"Large Neural Models' Self-Learning Symbolic Knowledge"
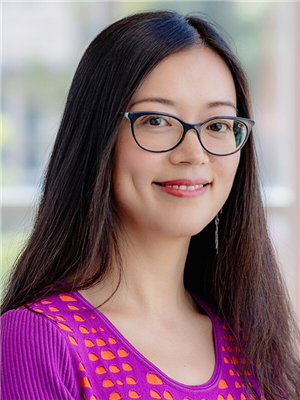
Abstract:
Recent large neural models have shown impressive performance on various data modalities, including natural language, vision, programming language and molecules. However, they still have surprising deficiency (near-random performance) in acquiring certain types of knowledge such as structured knowledge and action knowledge. In this talk I propose a two-way knowledge acquisition framework to make symbolic and neural learning approaches mutually enhance each other. In the first stage, we will elicit and acquire explicit symbolic knowledge from large neural models. In the second stage, we will leverage the acquired symbolic knowledge to augment and enhance these large models.
I will present three recent case studies to demonstrate this framework:
(1) The first task is to induce event schemas (stereotypical structures of events and their connections) from large language models by incremental prompting and verification [Li et al., ACL2023], and apply the induced schemas to enhance event extraction and event prediction.
(2) In the second task, we noticed that current large video-language models rely on object recognition abilities as a shortcut for action understanding. We utilize a Knowledge Patcher network to elicit new action knowledge from the current models and a Knowledge Fuser component to integrate the Patcher into frozen video-language models.
(3) In the third atask, we use large-scale molecule-language models to discover molecule subgraph structures ("building blocks") which contribute to blood brain barrier permeability in the kinase inhibitor family, and propose several candidate kinase inhibitor variants with improved ability to pass the blood brain barrier to accelerate better drug discovery. Then we can encode such graph pattern knowledge using lightweight adapter modules, bottleneck feed-forward networks that are inserted into different locations of backbone large molecule-language models.
Bio:
Heng Ji is a professor at Computer Science Department, and an affiliated faculty member at Electrical and Computer Engineering Department and Coordinated Science Laboratory of University of Illinois Urbana-Champaign. She is an Amazon Scholar. She is the Director of Amazon-Illinois Center on AI for Interactive Conversational Experiences (AICE). She received her B.A. and M. A. in Computational Linguistics from Tsinghua University, and her M.S. and Ph.D. in Computer Science from New York University. Her research interests focus on Natural Language Processing, especially on Multimedia Multilingual Information Extraction, Knowledge-enhanced Large Language Models, Knowledge-driven Generation and Conversational AI. She was selected as "Young Scientist" and a member of the Global Future Council on the Future of Computing by the World Economic Forum in 2016 and 2017. She was named as part of Women Leaders of Conversational AI (Class of 2023) by Project Voice. The awards she received include "AI's 10 to Watch" Award by IEEE Intelligent Systems in 2013, NSF CAREER award in 2009, PACLIC2012 Best paper runner-up, "Best of ICDM2013" paper award, "Best of SDM2013" paper award, ACL2018 Best Demo paper nomination, ACL2020 Best Demo Paper Award, NAACL2021 Best Demo Paper Award, Google Research Award in 2009 and 2014, IBM Watson Faculty Award in 2012 and 2014 and Bosch Research Award in 2014-2018. She was invited by the Secretary of the U.S. Air Force and AFRL to join Air Force Data Analytics Expert Panel to inform the Air Force Strategy 2030. She is the lead of many multi-institution projects and tasks, including the U.S. ARL projects on information fusion and knowledge networks construction, DARPA ECOLE MIRACLE team, DARPA KAIROS RESIN team and DARPA DEFT Tinker Bell team. She has coordinated the NIST TAC Knowledge Base Population task since 2010. She was the associate editor for IEEE/ACM Transaction on Audio, Speech, and Language Processing, and served as the Program Committee Co-Chair of many conferences including NAACL-HLT2018 and AACL-IJCNLP2022. She is elected as the North American Chapter of the Association for Computational Linguistics (NAACL) secretary 2020-2023. Her research has been widely supported by the U.S. government agencies (DARPA, NSF, DoE, ARL, IARPA, AFRL, DHS) and industry (Amazon, Google, Facebook, Bosch, IBM, Disney).
- June 28 (Wednesday), 17:00-18:00
Matthew Walter (Toyota Technological Institute at Chicago
)
Learning Better Ways to Measure and Move: Joint Optimization of
an Agent's Physical Design and Computational Reasoning
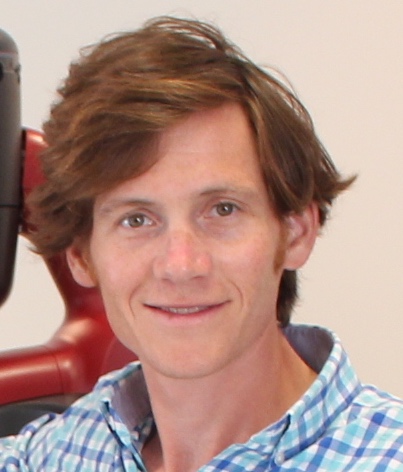
Abstract:
The recent surge of progress in machine learning foreshadows the
advent of sophisticated intelligent devices and agents capable of rich
interactions with the physical world. Many of these these advances
focus on building better computational methods for inference and
control---computational reasoning methods trained to discover and
exploit the statistical structure and relationships in their problem
domain. However, the design of physical interfaces through which a
machine senses and acts in its environment is as critical to its
success as the efficacy of its computational reasoning. Perception
problems become easier when sensors provide measurements that are more
informative towards the quantities to be inferred. Control policies
become more effective when an agent's physical design permits greater
robustness and dexterity in its actions. Thus, the problems of
physical design and computational reasoning are coupled, and the
answer to what combination is optimal naturally depends on the
environment the machine operates in and the task before it.
I will present learning-based methods that perform automated,
data-driven optimization over sensor measurement strategies and
physical configurations jointly with computational inference and
control. I will first describe a framework that reasons over the
configuration of sensor networks in conjunction with the corresponding
algorithm that infers spatial phenomena from noisy sensor readings.
Key to the framework is encoding sensor network design as a
differential neural layer that interfaces with a neural network for
inference, allowing for joint optimization using standard techniques
for training neural networks. Next, I will present a method that draws
on the success of data-driven approaches to continuous control to
jointly optimize the physical structure of legged robots and the
control policy that enables them to locomote. The method maintains a
distribution over designs and uses reinforcement learning to optimize
a shared control policy to maximize the expected reward over the
design distribution. I will then describe recent work that extends
this approach to the coupled design and control of physically
realizable soft robots. If time permits, I will conclude with a
discussion of ongoing work that seeks to improve test-time
generalization of the learned policies.
Bio:
Matthew R. Walter is an associate professor at the Toyota
Technological Institute at Chicago. His interests revolve around the
realization of intelligent, perceptually aware robots that are able to
act robustly and effectively in unstructured environments,
particularly with and alongside people. His research focuses on
machine learning-based solutions that allow robots to learn to
understand and interact with the people, places, and objects in their
surroundings. Matthew has investigated these areas in the context of
various robotic platforms, including autonomous underwater vehicles,
self-driving cars, voice-commandable wheelchairs, mobile manipulators,
and autonomous cars for (rubber) ducks. Matthew obtained his Ph.D.
from the Massachusetts Institute of Technology and the Woods Hole
Oceanographic Institution, where his thesis focused on improving the
efficiency of inference for simultaneous localization and mapping.
- June 29 (Thursday), 10:00-11:00
Angelica Lim (Simon Fraser University)
"Multimodal Machine Learning for Robot Social Intelligence and Empathy" (online presentation)
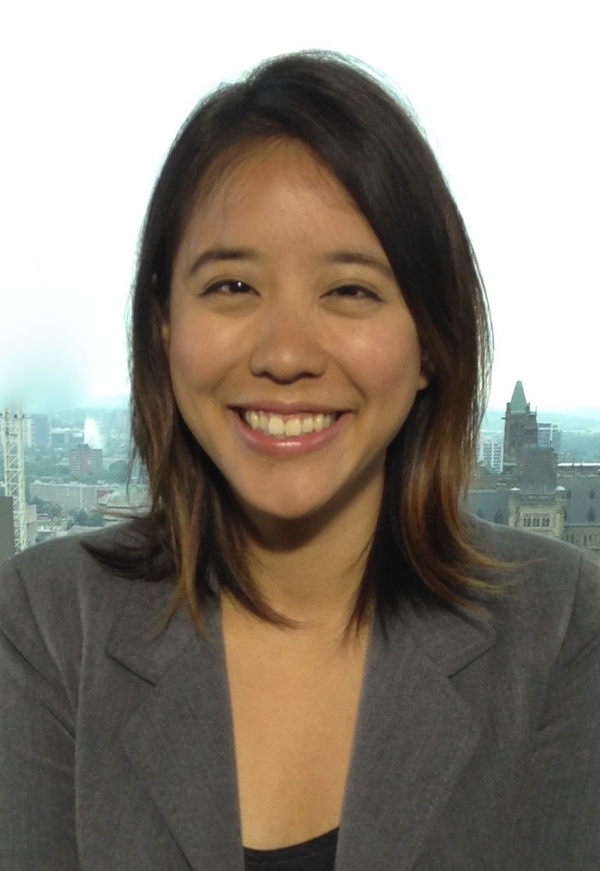
Abstract:
Interactive robots and voice user interfaces are moving us closer to
effortless, human-like interactions in the real world. In this talk, I
will discuss the opportunities and challenges in creating multimodal
machine learning systems that can analyze, detect and generate
non-verbal communication, including gestures, gaze, auditory signals,
and facial expressions. Specifically, I will discuss how we might
allow robots to understand human social signals (including emotions,
mental states, and attitudes) across cultures as well as to recognize
and generate expressions with diversity in mind.
Bio:
Dr. Angelica Lim is the Director of the Rosie Lab, and an Assistant
Professor in the School of Computing Science at Simon Fraser
University in Canada. Previously, she led the Emotion and Expressivity
teams for the Pepper humanoid robot at SoftBank Robotics. She received
her Ph.D. and Masters in Computer Science (Intelligence Science) from
Kyoto University, and a B.Sc. in Computing Science (Artificial
Intelligence Specialization) from Simon Fraser University. She has
been featured on the BBC, TEDx, hosted a TV documentary on robotics,
and was recently featured in Forbes 20 Leading Women in AI.
- June 29 (Thursday), 11:00-12:00
Albert Gu (Stanford University)
"Structured State Space Models for Deep Sequence Modeling"
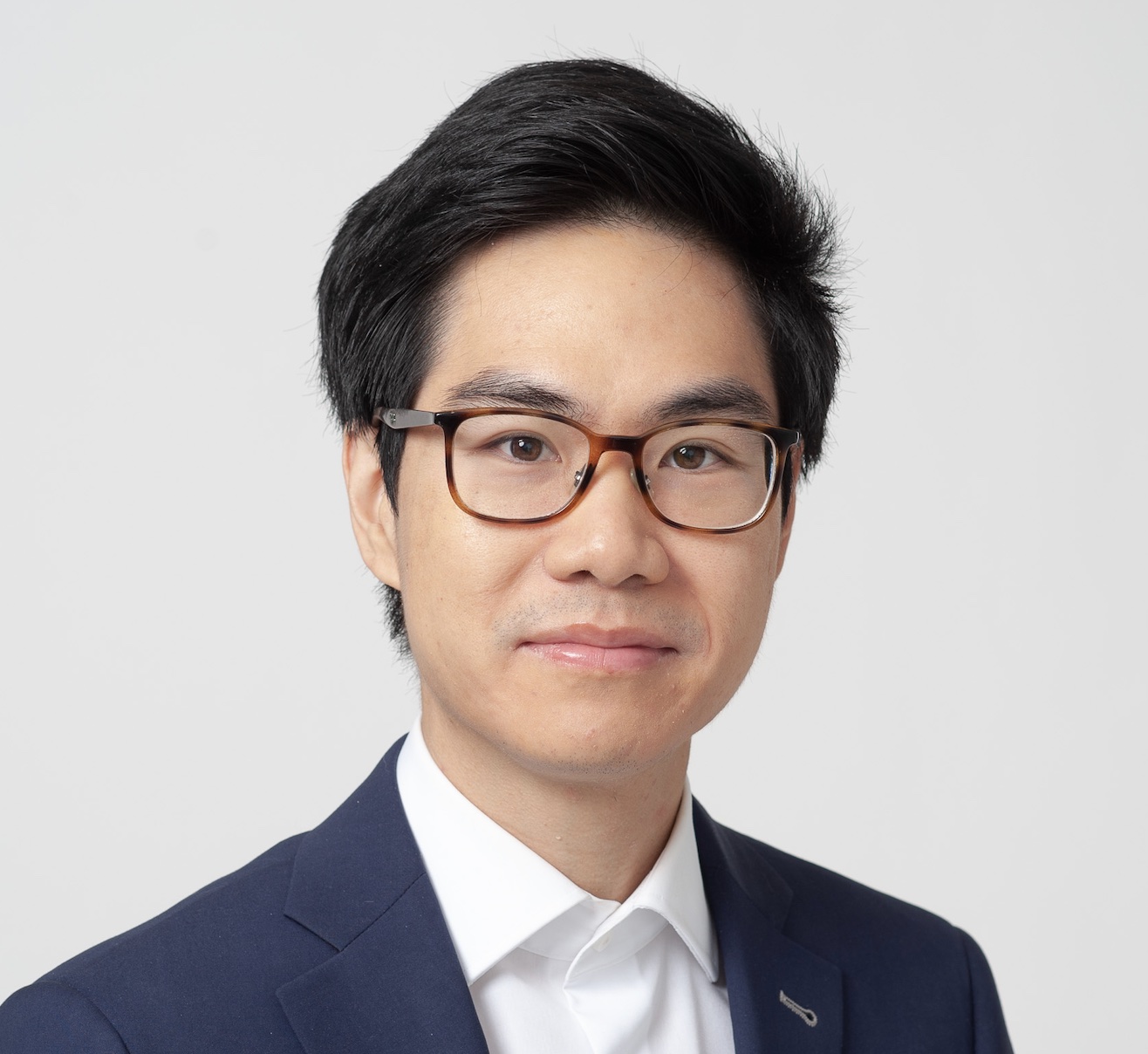
Abstract:
Substantial recent progress in machine learning has been driven by advances in sequence models, which form the backbone of deep learning models that have achieved widespread success across scientific applications. However, existing methods still have many drawbacks, including computational inefficiency and difficulty modeling more complex sequential data, such as when long dependencies are involved. As such, it remains of fundamental importance to continue to develop principled and practical methods for modeling sequences. This talk covers the structured state space approach to sequence modeling (S4), a flexible method that is theoretically grounded, computationally efficient, and achieves strong results across a variety of data modalities and applications. We will also discuss the relationship between SSMs and other deep sequence models, as well as several variations and applications of S4. Overall, this new family of methods provides effective and versatile building blocks for machine learning models, especially towards addressing general sequential data at scale.
Bio:
Albert is an incoming Assistant Professor of Machine Learning at Carnegie Mellon University. His research broadly focuses on theoretical and empirical aspects of deep learning, with a recent focus on new approaches for deep sequence modeling. He completed his PhD at Stanford University under the supervision of Christopher Re, and is currently working at DeepMind during a gap year.
Invited Talks:
- June 28 (Wednesday), 14:15-14:45
Yuta Nakashima (Osaka University)
"Concept discovery from an image dataset: Toward image representation with an emergent language"
Abstract:
Modeling deeper interactions among various concepts in visual and linguistic data is one of the key challenges to making artificial intelligence with a better capability of understanding images, videos, and text. Given the recent success in large language models (LLMs), we hypothesize that discrete representation of visual information, like a language, can mitigate the data greediness in training by simplifying possible combinations of concepts and with some help of LLMs. To this end, we are currently building an emergent language for visual representation. This talk introduces our first attempt toward this goal to automatically discover a set of concepts from an image classification dataset, which can be seen as a vocabulary of the language.
Bio:
Yuta Nakashima is an Associate Professor at the Institute for Datability Science, Osaka University. He received the B.E. and M.E. degrees in communication engineering and the Ph.D. degree in engineering from Osaka University, Osaka, Japan, in 2006, 2008, and 2012, respectively. From 2012 to 2016, he was an Assistant Professor at the Nara Institute of Science and Technology. His research interests include computer vision and machine learning and their applications.
- June 28 (Wednesday), 14:45-15:15
Yusuke Mukuta (University of Tokyo)
" On training and application of equivariant neural networks "
Abstract:
Equivariant neural networks are the neural network models that incorporate the prior knowledge about invariance into the model structure, which demonstrate good recognition accuracy owing to the invariance regularization. In this talk, we will present our resent work about the training method about the self-supervised learning on equivariant neural networks and the application of equivariance to the multiple time series forecasting.
Bio:
Yusuke Mukuta works as a Lecturer at the University of Tokyo, Japan. He obtained Ph. D in information science and technology from the University of Tokyo in 2018. From 2018 to 2020, he was an assistant professor at the University of Tokyo. He also works as a visiting researcher at the Riken AIP. His research interests include machine learning, computer vision and feature learning.
- June 28 (Wednesday), 16:30-17:00
Naoto Inoue (AI Lab in CyberAgent)
" Generative models for assisting graphic design"
Abstract:
Graphic design can be perceived as a corpus of document data characterized by a distinctive multimodal structure encompassing imagery, textual elements, spatial arrangements, and nuanced attributes such as typographic features. Establishing technologies for processing or generation of this complex data format is essential in facilitating collaborative design endeavors between human agents and artificial intelligence. This presentation will elucidate our recent endeavors towards the aforementioned objective, including layout generation, iterative design process modeling, and webpage colorization.
Bio:
Naoto Inoue is a Research Scientist at AI Lab in CyberAgent Inc. He obtained his Ph.D. at The University of Tokyo in 2021. His work focuses on generative models for computer vision, especially for graphic design.
- June 29 (Thursday), 13:30-14:00
Masanao Ochi (University of Tokyo)
"Integration of Linguistic and Citation Information for Science Foresight"
Abstract:
Identifying promising research as early as possible is vital to determine which research deserves investment. Additionally, developing a technology for automatically predicting future research trends is necessary because of increasing digital publications and research fragmentation. In previous studies, many researchers have predicted scientific indices using specially designed features for each index. However, this does not capture actual research trends. Developing a more integrated method to capture real research trends from various directions is necessary. Academic literature contains many data types, including language, citations, figures, tables, etc. Since the release of the Transformer model in 2017, there has been an enthusiastic research focus on models that integrate data in a multimodal fashion. This talk will highlight recent efforts to integrate linguistic and network information to predict academic impact.
Bio:
Masanao Ochi is a project researcher at the University of Tokyo. His research mainly focuses on network analysis and representation learning for networks. He received his Ph.D. from the University of Tokyo in 2017.
- June 29 (Thursday), 14:00-14:30
Ryo Ueda (University of Tokyo)
"On the Word Boundaries of Emergent Languages"
Abstract:
Emergent Languages are artificial communication protocols arising among agents. However, it is not obvious whether such a simulated language would have the same properties as natural language. In our recent paper, we tested if they satisfy Harris's Articulation Scheme (HAS), which states that word boundaries can be obtained solely from phonemes in natural language. We adopted HAS-based word segmentation and verified whether emergent languages have meaningful word segments. The experiment suggested they do not have, although they met some preconditions for HAS. We discovered a gap between emergent and natural languages to be bridged, indicating that the standard signaling game satisfies prerequisites but is still missing some necessary ingredients.
Bio:
Ryo Ueda received his bachelor's degree in Information Science from the University of Tokyo in 2021 and his M.S. in Computer Science from the Graduate School of Information Science and Technology, the University of Tokyo, in 2023. He is currently a doctoral student in the same department and a JSPS Research Fellow (DC1) from 2023. He is interested in emergent communication.
- June 29 (Thursday), 14:30-15:00
Katsuhiko Hayashi (Hokkaido University)
"Distributed representations of compositional structures"
Abstract:
Distributed representations are known to be useful for modeling large-scale relational learning and classification tasks. In this talk, I will discuss methods to represent structured data in low-dimensional vector space. I will especially focus on techniques using circular convolution for encoding compositional structures into low-dimensional vector representations.
Bio:
Katsuhiko Hayashi received the Ph.D. degree in engineering from Nara Institute of Science and Technology, Japan, in 2013. From 2013 to 2016, he was a researcher at Nippon Telegraph and Telephone Corporation (NTT). He is currently an associate professor at the Faculty of Information Science and Technology, Hokkaido University. His research interests include machine learning and formal language technologies for natural language processing.
- June 29 (Thursday), 16:15-16:45
Kazu Ghalamkari (RIKEN)
"Tensor Factorization Using Interaction Modeling"
Abstract:
Tensor low-rank decomposition approximates tensors with rank-reduced
tensors; however, the cost function is non-convex. Thus, it is
difficult to obtain the global optimum. In this talk, we propose
interactions between modes as an alternative concept to rank for
tensor decomposition. We regard tensors as parameterized mass
probability functions whose sample space is the index set. We can then
reduce interactions by restricting their parameters. The subspaces of
interaction-reduced tensors are flat. Thus, we eliminate the
non-convexity of the cost function. This enables the natural gradient
method to rapidly provide the global optimum relative to the
Kullback–Leibler divergence from the input tensor. Moreover, we
introduce interaction representation as a diagram that intuitively
describes existing and absent interactions among modes. By converting
the diagram to tensor networks, we also reveal a non-trivial
relationship between the proposal and traditional tensor
decompositions.
Bio:
Kazu Ghalamkari is a postdoctoral researcher with RIKEN AIP. He
obtained his PhD in Informatics from SOKENDAI, Japan, in 2023.
Previously, he worked as a full-time researcher at Hitachi Ltd. He is
developing machine learning methodologies that leverage insights from
physics. His recent interests lie in information geometry,
energy-based models, and tensor decomposition.
- June 29 (Thursday), 16:45-17:15
Go Irie (Tokyo University of Science)
"Seeing through Sounds: Visual Scene Understanding from Acoustic Signals"
Abstract:
Sound provides a multitude of informative cues about surrounding environments. In this talk, we will introduce our recent projects for recognizing visual scenes from acoustic signals. Specifically, we will present our efforts aimed at scene recognition, human pose estimation, and action recognition.
Bio:
Go Irie is an associate professor at Tokyo University of Science, Japan. He was a research scientist at NTT Corporation, Japan, from 2006 to 2022, and a visiting researcher at Columbia University from 2012 to 2013. His research interests include pattern recognition, machine learning and media understanding.
|